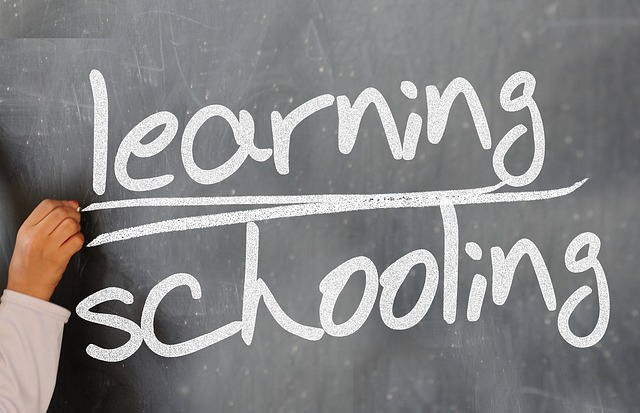
Statistics may not be the hardest form of mathematics, but it is probably the most finicky. Moreover, the fact that it is vitally important in many fields means that it needs to be understood by people who otherwise would want nothing to do with mathematics. It is no wonder that when people talk about statistics, they tend to be wrong. One of the most relevant examples of this is adjusting test scores according to a normal distribution, which is common in some majors. Relying on faulty assumptions, curving based on the normal distribution creates a system where knowing who your classmates will be is almost as important as knowing the material, and this only impedes learning.
Grading tests based on a normal curve is built on a faulty assumption that test scores are supposed to be based on a normal distribution, where a large percentage of the class should be centered around the average score. There is no real reason to assume this. It comes from a misunderstanding of the central limit theorem. When a large enough sample is taken from any population, we expect the sample mean to follow a normal distribution, not the elements of the sample itself. To properly apply the central limit theorem to test scores, it would require drawing a random sample of all students in any section of a given class, giving them a test, averaging this sample’s scores and then repeating this process multiple times. Only by looking at these sample means can we see a normal distribution.
Even if it did not rely on bad mathematics, it is clear that this type of curve can create situations which are beneficial to no one. Suppose I am an average student and I have a friend called S who is brilliant. We want to take a class which is offered in two sections. Assume everyone else in this class is as good as me. If I take the class with S, there will likely be an upper limit on how well I can do, because I will always be graded in comparison to him. As a result, ensuring I do not share a class with him can do more for my grade than actually studying. Note that these problems are not unique to grading on a normal distribution, but they apply to every grading scale that strictly requires a certain proportion of students to get each possible grade.
This is not only a problem in classrooms; a faulty understanding of statistics can undermine businesses as well. Microsoft once employed a “stack ranking” evaluation system where the highest-working proportion of any group received bonuses and the lowest proportion could be fired. Intended to trim excess workers, it in fact made good workers unlikely to work together, as they might look worse by comparison. Moreover, departments were forced to label their lowest performers, who would receive no bonuses and even possibly be fired, simply because they did not match up with those who were better. This resulted in a destructive culture. According to one employee in a July 2012 Vanity Fair article, “It leads to employees focusing on competing with each other rather than competing with other companies.”
Microsoft has since gotten rid of the “forced curve” element of its stack ranking system, now lifting the limits on the proportion of employees that can earn a certain score. Since the changes, Microsoft has seen drastic improvement, including a substantial increase in share price. In the business world, as in the classroom, using preconceived assumptions about how people should perform in relation to each other will always shift the focus away from producing good work and ideas.
Andrew Langen is a junior majoring in economics and math.
Featured image courtesy Pixabay geralt